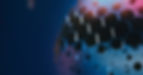
NO MAGIC,
JUST SCIENCE.
Our goal is to build a machine that reads and processes text like human would do. This requires a combination of cognitive psychology, semantic computing, and machine learning. Headai approach emulates the human way to learn: According to the cognitive psychology of learning, our thinking is based on conceptual representations of our observations, experiences, and relations between these concepts. Phenomena when the structure (concepts or relationships) change is called learning.
​
Headai’s AI learns the work context via general unstructured content and teaching done by humans. In phase 1 it learns the basic semantics of relations of the working context. The learning in this phase follows the ideas of unsupervised learning. In phase 2 the process applies reinforcement learning: the user teaches it by evaluating its performance. The general content for first phase teaching can be e.g. text documents, databases, conceptual maps, graphs, etc. This means the AI can be taught to handle very different tasks.
What are Headai’s ML & NLP benefits compared to Deep Learning applications?
Green AI
Uses only a fraction of energy compared to Deep Learning solutions.
Cognitive reasoning
Enables complex tasks like reasoning with controversial and/or incomplete information
Expalinability
The AI results can easily be explained – there are no black boxes.
Ready to operate
Operates straight away, even with insufficient data and changing conditions. DL applications are sensitive to changes and require a massive amount of training.
25M
Job ads
Worldwide
800k
Course Descriptions
10M
Scientific papers

Digital Self – The Core Model Behind Simulations
An interoperable machine learning data model of any entity’s skill assets. It is based on Self-Organizing Maps (SOM) type of unsupervised learning, which keeps it up-to-date. A digital self can model e.g. skills supply, skills demand, skills forecasts, an individual’s professional profile, educational curriculums, SDGs (UN’s Sustainable Development Goals), and more. Everything can be simulated against each other. Headai Digital Self is interoperable with major labor market standards like ISCO (UN), ESCO (EU), SOC, and O*NET (US). It enables cognitively complex tasks like reasoning with controversial and/or incomplete information (Deep Learning models won’t enable cognitively complex operations). The training of the model can be done in multiple ways with sources like: Global Labour Market Standards,
Global Business Information System, Economical News & Financial Media, Job Ads & Work Foresight Reports, Patents, Research & Innovations.

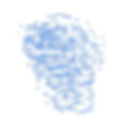
Headai Dynamic Ontology
The dynamic machine learning model for words, semantics, and meanings is based on self-organizing maps (SOM) type of unsupervised learning. It can be used to build always up-to-date and detailed language models for different situations.
It is based on terabytes of open textual data acquired from the real world: scientific articles, reports, curriculums, course descriptions, job descriptions, and job vacancies. Enables cognitively complex tasks such as reasoning with controversial and/or incomplete information (most deep learning models do not allow cognitively complex functions). Outperforms DL models in computational speed and performance relative to computational capacity.
The general language model is a core component in Headai technology.
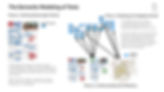
SCIENTIFIC ARTICLES
Some of our team’s scientific publications
-
Ketamo, H. (2015). Taking Printed Books into Internet of Things.
In Berntzen & Böhm (eds.), CENTRIC 2015, The Eighth International Conference on Advances in Human oriented and Personalized Mechanisms, Technologies, and Services. November 15 - 20, 2015, Barcelona, Spain, pp. 5-11. -
Ketamo, H. (2014). Learning Fingerprint: Adaptive Tutoring for MOOCs.
In Proceedings of World Conference on Educational Multimedia, Hypermedia and Telecommunications. Jun 23, 2014, Tampere, Finland, pp. 2349–2358. -
Ketamo, H. (2013). Agents and Analytics.
In proceedings of 5th International Conference on Agents and Artificial Intelligence, vol2 (ICAART 2013), 15-18 February 2013, Barcelona, Spain, pp. 377-383. -
Ketamo, H., Nurmi, C. & Kallama, K. (2011). User Generated AI in Sports Education.
In proceedings of the 5th European Conference on Games Based Learning. 20-21 October 2011, Athens, Greece, pp. 306-314. -
Ketamo, H. (2011). Sharing Behaviors in Games and Social Media.
International Journal of Applied Mathematics and Informatics, vol. 5(1), pp. 224-232. -
Ketamo, H. (2009). Self-organizing content management with semantic neural networks.
In Recent Advances in Neural Networks: Proceedings of the 10th WSEAS International Conference on Neural Networks (NN’09), Prague, Czech Republic, 23-25.3. -
Ketamo, H. (2008). Cost Effective Testing with Artificial Labour.
In proceedings of 2008 Networked & Electronic Media Summit. Saint-Malo, France, 13-15.10.2008, pp.185-19